views
These silos emerge as a natural consequence of the diverse sources and proprietary systems that generate data in the field. Clinical data, genomic sequences, proteomic datasets, and imaging files are often stored separately, each following its own standards, formats, and storage mechanisms. While this setup might suit individual teams, the lack of interconnectedness leads to fragmented data ecosystems that are difficult to integrate and analyze holistically.
The impact of data silos on data quality is profound. When datasets remain isolated, inconsistencies and redundancies become unavoidable. For example, one research team may collect patient data using different units of measurement or variable names than another, making harmonization a labor-intensive and error-prone process. Additionally, crucial metadata contextual information that provides meaning to raw data is often incomplete or missing altogether, further reducing the usability of siloed datasets.
Source Url
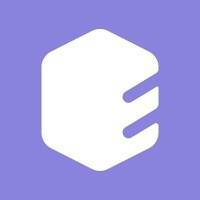
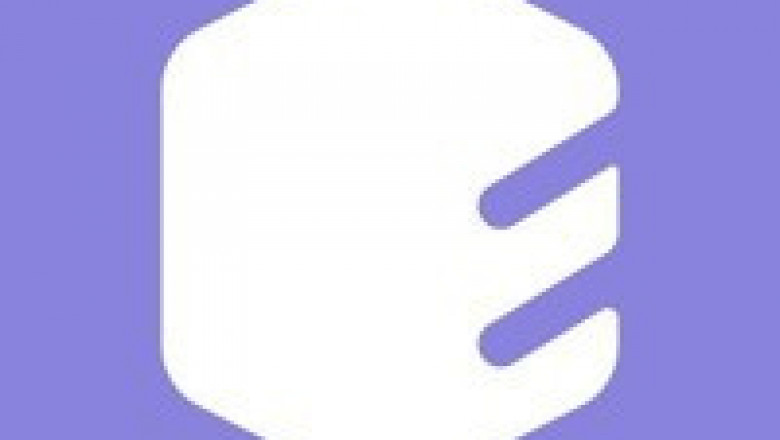

Comments
0 comment