views
Machine learning (ML) is changing the way researchers identify potential drug candidates, predict molecular interactions, and optimize clinical trials. ML models are accelerating discovery timelines and increasing success rates in drug discovery. However, the success of these models relies on their design and how good they are at predicting potential drugs and their targets.
To judge the reliability of an ML model, the right evaluation metrics are essential. In drug discovery, where small decisions can alter workflow trajectories, selecting the right metrics is critical. Standard metrics like accuracy or mean squared error (MSE), though useful in generic ML tasks, often fall short when it comes to biopharma.
Biopharma deals with imbalanced datasets with far more inactive compounds than active ones. This imbalance can render traditional metrics misleading. For example, a model might achieve high accuracy by predicting the majority class (inactive compounds) while failing to identify active ones, which are the primary targets in drug discovery.
Additionally, biopharma deals with rare but critical events, such as adverse drug reactions or gene expression outliers in omics data. Predicting these events needs evaluation methods that emphasize sensitivity and the ability to capture outliers, rather than rewarding overall correctness. Furthermore, the data in biopharma is often multi-modal, integrating diverse sources like chemical properties, biological assays, and clinical trial outcomes. This complexity requires metrics that can assess model performance across heterogeneous inputs and outputs.
The limitations of conventional metrics highlight the need for performance metrics customized to the specific needs of biopharma. Metrics that account for imbalanced datasets, multi-modal inputs, and rare-event detection are a must for ensuring that ML models provide helpful insights. By using appropriate evaluation methods for biopharma-specific challenges, researchers can interpret their model outputs better, ultimately driving more effective drug discovery processes.
Source: https://www.elucidata.io/blog/evaluation-metrics-for-ml-models-in-drug-discovery
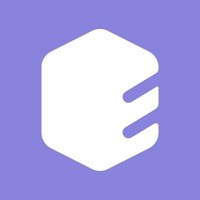
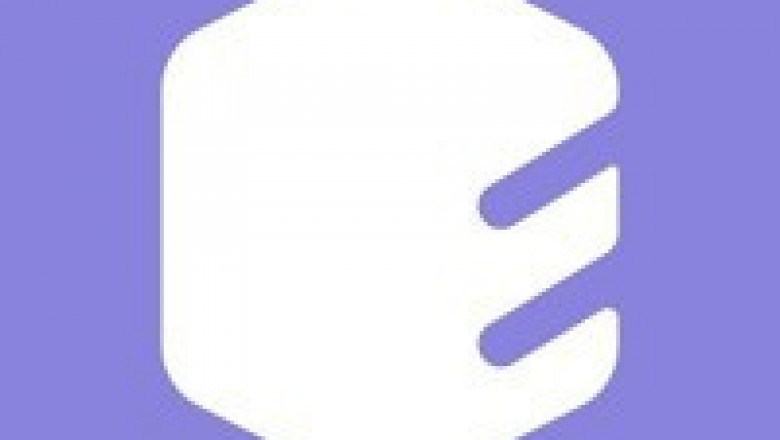

Comments
0 comment