views
Artificial Intelligence (AI) and Machine Learning (ML) are revolutionizing industries, transforming how we process data, make decisions, and interact with technology. AI, driven by ML, is shaping the future by enabling systems to learn from data, recognize patterns, and make intelligent decisions without human intervention. This article explores how AI in ML is driving innovation, its applications, benefits, challenges, and what the future holds.
Understanding AI and Machine Learning
AI refers to the broader concept of machines performing tasks that typically require human intelligence, such as reasoning, problem-solving, and decision-making. Machine Learning, a subset of AI, involves algorithms that allow computers to learn and improve from experience without being explicitly programmed.
ML models learn by analyzing vast datasets, identifying trends, and making predictions. This ability to self-improve makes ML a crucial part of AI's evolution, leading to advancements in automation, predictive analytics, and intelligent decision-making.
How AI is Transforming Machine Learning
AI enhances ML by improving accuracy, efficiency, and scalability. Some key ways AI is transforming ML include:
- Automated Feature Engineering – AI helps in selecting the most relevant data features for training ML models, reducing the need for manual intervention.
- Deep Learning Advancements – AI-powered deep learning models simulate human neural networks, leading to breakthroughs in image recognition, natural language processing, and robotics.
- Real-Time Decision Making – AI-driven ML enables systems to process vast amounts of data instantly, allowing industries like finance and healthcare to make split-second decisions.
- Explainable AI (XAI) – XAI helps interpret ML models, making them more transparent and understandable to users.
Key Applications of AI in Machine Learning
AI-powered ML is widely used across various industries, offering innovative solutions that enhance efficiency and accuracy. Some prominent applications include:
1. Healthcare and Medical Diagnosis
AI-driven ML models analyze medical images, detect diseases, and predict patient outcomes with high accuracy. For example, AI-powered radiology systems assist doctors in identifying tumors in MRI and CT scans.
2. Finance and Fraud Detection
Financial institutions use AI to analyze transaction patterns, detect fraudulent activities, and automate risk assessments. AI-driven chatbots also provide customer support and financial advice.
3. Autonomous Vehicles
Self-driving cars rely on AI-powered ML algorithms to process sensor data, recognize obstacles, and navigate safely. AI enhances real-time decision-making, making autonomous driving a reality.
4. E-Commerce and Personalized Recommendations
AI-driven ML models analyze customer behavior to provide personalized product recommendations. Platforms like Amazon and Netflix use AI to enhance user experiences.
5. Cybersecurity and Threat Detection
AI-powered ML systems detect cyber threats by analyzing network patterns, identifying anomalies, and responding to security breaches in real time.
6. Natural Language Processing (NLP) and Chatbots
AI-driven NLP enables chatbots and virtual assistants like Siri and Alexa to understand and respond to human queries with high accuracy, enhancing customer interactions.
Benefits of AI in Machine Learning
AI-driven ML offers numerous advantages, including:
- Improved Accuracy – AI enhances ML models, reducing errors and improving predictive capabilities.
- Automation of Repetitive Tasks – AI-powered ML automates data processing, freeing up human resources for complex tasks.
- Enhanced Decision-Making – AI-driven analytics provide data-driven insights, helping businesses make informed decisions.
- Scalability – AI enables ML models to handle vast amounts of data, making them suitable for large-scale applications.
- Continuous Learning – AI allows ML models to self-improve, adapting to new data and evolving over time.
Challenges of AI in Machine Learning
Despite its potential, AI-driven ML faces several challenges:
- Data Privacy and Security – AI models rely on vast amounts of data, raising concerns about data privacy and ethical use.
- Bias in AI Models – AI-driven ML can inherit biases from training data, leading to biased decision-making.
- High Computational Costs – Training AI-powered ML models requires significant computing resources and energy consumption.
- Lack of Explainability – Some AI-driven ML models operate as "black boxes," making it difficult to understand their decision-making process.
- Regulatory and Ethical Concerns – AI in ML raises ethical concerns about job displacement, surveillance, and potential misuse.
The Future of AI in Machine Learning
The future of AI in ML looks promising, with advancements in:
- Federated Learning – AI will enable ML models to train on decentralized data sources while preserving privacy.
- Quantum AI – The integration of quantum computing with AI will significantly enhance ML capabilities, solving complex problems faster.
- AI-Augmented Creativity – AI-driven ML will revolutionize creative fields like art, music, and content creation.
- AI in Edge Computing – AI-powered ML will be deployed at the edge, enabling real-time decision-making in IoT and smart devices.
- Ethical AI Development – Organizations will focus on making AI-driven ML more transparent, fair, and responsible.
Conclusion
AI in Machine Learning is powering the future of intelligence by driving innovation across various industries. From healthcare and finance to cybersecurity and autonomous systems, AI-powered ML is transforming how we process and interpret data. While challenges such as data privacy and bias remain, ongoing advancements in AI-driven ML will continue to unlock new possibilities, making technology smarter, more efficient, and more accessible.
As AI and ML evolve, their impact on society will only grow, shaping a future where intelligent machines complement human intelligence, driving progress and innovation across all domains.
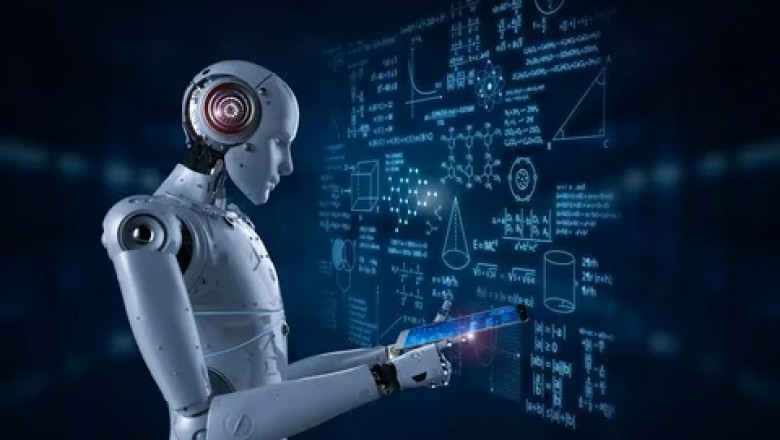

Comments
0 comment