views
The global Machine Learning Operations (ML Ops) market has witnessed substantial growth in recent years. Valued at USD 1.23 billion in 2023, the market is expected to continue its upward trajectory, with an impressive compound annual growth rate (CAGR) of 20.36% through 2029. This rapid expansion reflects the growing adoption of machine learning (ML) and artificial intelligence (AI) technologies across various industries. One of the key catalysts for this growth is the integration of ML Ops with cloud platforms, which enhances scalability, flexibility, and overall efficiency in managing the machine learning lifecycle.
Request For Sample Copy of Report For More Detailed Market insight: https://www.techsciresearch.com/sample-report.aspx?cid=7925
In this comprehensive report, we explore the key trends, drivers, challenges, and opportunities that are shaping the ML Ops market, along with a detailed competitive analysis and future outlook. As organizations increasingly seek to streamline their machine learning workflows, the role of ML Ops has become more prominent, facilitating faster deployment, continuous model monitoring, and automated maintenance. With the evolving landscape of AI and ML technologies, the demand for sophisticated and efficient ML Ops solutions is expected to surge in the coming years.
Browse over XX Market data Figures spread through XX Pages and an in-depth TOC on the "Global ML Ops Market.”
Industry Key Highlights
The ML Ops market is witnessing several developments that highlight the increasing importance of this technology. Key highlights from the global ML Ops market include:
- Integration with Cloud Platforms: As businesses adopt cloud computing for its scalability and cost efficiency, integrating ML Ops with cloud platforms has become a common practice. This integration supports the end-to-end machine learning lifecycle, from data ingestion to model deployment and monitoring.
- Increasing Adoption of AI and ML Technologies: Industries such as healthcare, finance, manufacturing, and retail are increasingly relying on AI and ML to drive innovation and optimize their operations. This growing demand for AI solutions is fueling the need for robust ML Ops platforms that streamline model deployment and ensure seamless integration with other business processes.
- Cloud Segment Dominance: The cloud deployment model is poised to dominate the ML Ops market, driven by its cost-effectiveness and flexibility. The ability to scale resources dynamically without investing in expensive on-premises infrastructure is a major factor contributing to the growth of the cloud-based ML Ops market.
- Asia-Pacific as the Fastest Growing Region: The Asia-Pacific region is emerging as the fastest-growing market for ML Ops, with countries like China, India, Japan, and South Korea leading the way in AI adoption and digital transformation initiatives.
- Robust Competitive Landscape: Major players such as IBM Corporation, Microsoft Corporation, Alphabet Inc., Amazon Web Services (AWS), and DataRobot are competing in the global ML Ops market. These companies are continuously enhancing their product offerings, with investments in research and development (R&D) and strategic partnerships playing a key role in market expansion.
Browse over XX Market data Figures spread through XX Pages and an in-depth TOC on the "Global ML Ops Market.” @https://www.techsciresearch.com/report/ml-ops-market/7925.html
Emerging Trends in the ML Ops Market
As the ML Ops market continues to expand, several emerging trends are shaping its future. These trends highlight the increasing sophistication of ML operations and the growing reliance on cloud technologies and automation.
1. AI-Driven Automation and Continuous Learning
One of the key trends driving the ML Ops market is the integration of AI-driven automation into the machine learning lifecycle. Automation of tasks such as data preprocessing, model training, and performance monitoring helps organizations accelerate the development and deployment of machine learning models. With the increasing volume and complexity of data, AI-driven tools are becoming essential for managing and optimizing the ML pipeline. These tools not only improve operational efficiency but also enable continuous learning by allowing models to update and retrain automatically based on new data.
2. Edge Computing Integration
Another emerging trend in the ML Ops market is the integration of edge computing with ML Ops platforms. Edge computing allows data to be processed closer to the source of generation, reducing latency and enhancing real-time decision-making. By combining ML Ops with edge computing, organizations can deploy machine learning models on edge devices, enabling faster and more efficient processing of data in real-time. This trend is particularly relevant for industries such as manufacturing, healthcare, and autonomous vehicles, where low-latency and real-time data processing are critical.
3. Model Governance and Compliance
As machine learning models are increasingly being adopted for business-critical applications, the need for robust model governance and regulatory compliance has become more prominent. ML Ops solutions are evolving to incorporate tools for model versioning, auditing, and monitoring, ensuring that models are transparent, explainable, and compliant with regulatory standards. This trend is particularly important in industries like healthcare, finance, and government, where strict regulatory requirements govern the use of AI and ML technologies.
4. Serverless Architectures
Serverless computing is gaining traction in the ML Ops market due to its ability to simplify the deployment and scaling of machine learning models. Serverless architectures eliminate the need for managing servers, enabling organizations to focus on developing and deploying machine learning models without worrying about underlying infrastructure. This flexibility allows businesses to scale their ML operations more easily and reduce operational overhead, making it an attractive option for organizations of all sizes.
5. Democratization of ML and AI
With advancements in cloud computing and the rise of low-code/no-code platforms, machine learning and AI technologies are becoming more accessible to non-technical users. This trend, known as the democratization of AI, is expanding the ML Ops market by allowing businesses to leverage machine learning capabilities without requiring specialized expertise. By simplifying the deployment and management of machine learning models, these platforms empower a wider range of organizations to implement AI-driven solutions and benefit from automation.
Drivers of the ML Ops Market
The growth of the ML Ops market is driven by several key factors, including the increasing adoption of machine learning technologies, the need for more efficient AI model deployment, and the growing importance of scalability and flexibility in managing ML workflows.
1. Increasing Adoption of Machine Learning
As machine learning becomes a core component of digital transformation strategies, the demand for ML Ops solutions is rising. Businesses are looking for ways to deploy machine learning models at scale, manage their lifecycle, and ensure optimal performance. ML Ops provides a structured approach to managing the ML pipeline, ensuring that models are developed, deployed, and monitored efficiently.
2. Growing Demand for AI-Powered Solutions
Industries such as healthcare, finance, retail, and manufacturing are increasingly relying on AI and ML to drive innovation and improve operational efficiency. As the adoption of AI-powered solutions grows, the need for robust ML Ops platforms that can manage the complexities of AI models becomes more apparent. ML Ops platforms enable businesses to automate workflows, scale resources, and monitor model performance, helping them extract maximum value from their AI investments.
3. Cloud Adoption and Scalability
The rise of cloud computing has been a significant driver of the ML Ops market. Cloud platforms offer the scalability and flexibility needed to manage large-scale ML workflows, making them an ideal solution for organizations looking to deploy machine learning models without the need for expensive on-premises infrastructure. Cloud-based ML Ops platforms enable businesses to scale resources up or down based on demand, optimizing costs while maintaining high levels of performance.
4. Need for Real-Time Data Processing
With the increasing amount of data generated by businesses, there is a growing need for real-time data processing to support AI-driven decision-making. ML Ops platforms that integrate with edge computing and cloud technologies enable organizations to process data in real-time, allowing them to make faster, more informed decisions. This is particularly important in industries such as manufacturing, healthcare, and finance, where real-time data is critical for maintaining operations and ensuring customer satisfaction.
5. Regulatory Compliance and Data Governance
As the use of AI and ML technologies grows, so does the need for regulatory compliance and data governance. ML Ops platforms are evolving to include features such as model versioning, monitoring, and auditing, ensuring that machine learning models are transparent, explainable, and compliant with industry regulations. This trend is particularly relevant in sectors like healthcare, finance, and government, where strict regulations govern the use of AI and ML.
Competitive Analysis
The competitive landscape of the global ML Ops market is dynamic and rapidly evolving. Major players in the market include:
- IBM Corporation: IBM is a key player in the ML Ops market, offering a range of AI and machine learning solutions through its Watson AI platform. The company focuses on providing end-to-end ML Ops capabilities, including model training, deployment, and monitoring.
- Microsoft Corporation: Microsoft offers Azure Machine Learning, a cloud-based platform that provides comprehensive ML Ops tools. The platform supports the entire machine learning lifecycle, from model development to deployment and monitoring, and integrates with other Azure services for enhanced scalability and performance.
- Alphabet Inc. (Google Cloud): Google Cloud’s AI and machine learning solutions are widely used in the ML Ops market. The company’s Vertex AI platform provides a suite of tools for managing the ML pipeline, including model training, deployment, and monitoring.
- Amazon Web Services (AWS): AWS provides a range of ML Ops services through its Amazon SageMaker platform. SageMaker enables businesses to build, train, and deploy machine learning models at scale, with built-in tools for monitoring and model optimization.
- DataRobot: DataRobot offers an enterprise AI platform that simplifies the deployment and management of machine learning models. The company’s ML Ops tools automate the machine learning lifecycle, making it easier for businesses to deploy AI models in production environments.
These companies, along with other players in the market, are continually enhancing their product offerings through strategic acquisitions, partnerships, and investments in R&D to stay competitive in the rapidly growing ML Ops market.
Future Outlook
The ML Ops market is expected to continue its rapid growth in the coming years, driven by the increasing adoption of machine learning and AI technologies across industries. As businesses seek to optimize their ML workflows and scale their operations, the demand for cloud-based ML Ops solutions will continue to rise. The integration of AI, cloud computing, and automation will play a crucial role in shaping the future of ML Ops, enabling organizations to deploy and manage machine learning models more efficiently and at scale.
In addition to cloud-based solutions, the rise of edge computing, serverless architectures, and AI-driven automation will create new opportunities for ML Ops platforms. As organizations increasingly look for ways to leverage real-time data and streamline their AI operations, the ML Ops market will continue to evolve, offering businesses the tools and capabilities they need to stay competitive in an AI-driven world.
Customers can also request 10% free customization in this report.
10 Benefits of the ML Ops Research Report
- Comprehensive Market Insights: The report offers a detailed analysis of the ML Ops market, including key trends, drivers, and challenges that are shaping the industry.
- Competitive Landscape: The report provides a thorough overview of the competitive landscape, highlighting the key players and their market share, strategies, and product offerings.
- Growth Opportunities: The report identifies emerging trends and growth opportunities in the ML Ops market, helping businesses make informed investment decisions.
- Regional Analysis: The report offers a detailed regional analysis, highlighting the fastest-growing regions and their potential for ML Ops adoption.
- Technological Advancements: The report explores the latest technological advancements in ML Ops, including AI-driven automation, cloud integration, and edge computing.
- Market Forecast: The report provides a market forecast for the ML Ops industry, helping businesses understand the future growth trajectory and potential challenges.
- Deployment Models: The report analyzes different deployment models, including cloud, on-premises, and hybrid, and their impact on the ML Ops market.
- Regulatory Insights: The report provides insights into regulatory requirements and data governance issues related to ML Ops, especially in highly regulated industries.
- Investment Opportunities: The report identifies key areas where investors can capitalize on growth opportunities in the ML Ops market.
- Comprehensive Data: The report provides in-depth data on market size, trends, and projections, offering valuable insights for decision-makers.
Conclusion
The ML Ops market is poised for rapid growth in the coming years, driven by the increasing adoption of machine learning and AI technologies across industries. The integration of ML Ops with cloud platforms, the rise of AI-driven automation, and the growing need for scalable and flexible solutions are key factors contributing to this growth. As the market evolves, businesses will continue to seek efficient ways to deploy, manage, and scale their machine learning models, creating significant opportunities for ML Ops providers. The future outlook for the ML Ops market is promising, with increasing demand for cloud-based solutions, edge computing, and AI-driven capabilities driving innovation and growth.
Customers can also request 10% free customization in this report.
Contact US:
Techsci Research LLC
420 Lexington Avenue, Suite 300,
New York, United States- 10170
Tel: +13322586602
Email: sales@techsciresearch.com
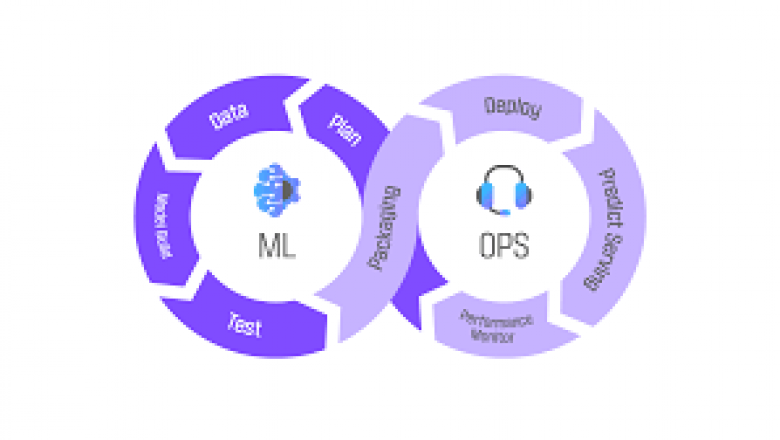

Comments
0 comment