views
Authors:
Dr Samanta, Assistant Professor, IIIT Allahabad
Ananya, PhD, Department of Applied Sciences, IIIT-Allahabad
Sarfraz Anwar, PhD, Department of Applied Sciences, IIIT Allahabad
Polypharmacy, the use of multiple drugs simultaneously, poses significant risks of adverse interactions. To mitigate these risks, improved AI-driven frameworks are essential for pre-dicting side effects. While research has utilized machine learning models like Artificial Neu-ral Networks and XGBoost to assess drug-drug and drug-protein interactions, protein-protein interactions remain unincorporated in datasets. Addressing this gap could enhance prediction accuracy, highlighting the need for further development of AI and ML models in polyphar-macy side-effect prediction.
In today's rapidly advancing field of modern medicine, the practice of prescribing multiple medications at once—referred to as polypharmacy—has become increasingly common, especially for individuals undergoing chronic or complex health conditions. While intake of more than one drug is essential for disease management, it also introduces a significant risk: the possibility of harmful drug interactions leading to serious or even life-threatening side effects. As a result, predicting these interactions has become a crucial focus in healthcare, driving the development of advanced AI and machine learning models designed to anticipate these dangers.
Traditionally, these models have been centered on understanding either drug-drug or drug-protein interactions, utilizing extensive datasets to identify potential risks. However, as our understanding of cellular biology deepens, it’s evident that these models may be missing a critical component: protein-protein interactions (PPIs). Proteins don’t function in isolation; they interact with other proteins, forming complex networks that are essential for biological processes. Disruptions in these networks—whether due to disease or drug effects—can lead to a cascade of unintended consequences that traditional models might overlook. By incorporating PPI data into our current frameworks, we’re on the cusp of a significant breakthrough in predicting polypharmacy side effects, which could result in safer, more personalized treatments, bringing us closer to the goal of truly individualized medicine.
Integrating PPIs into AI/ML Models
Incorporating protein-protein interactions (PPIs) into AI and machine learning models offers a promising way to enhance the accuracy of predicting side effects from polypharmacy. By taking into account the intricate network of protein interactions within cells, these models could more accurately forecast how a combination of drugs might disrupt normal cellular processes. For instance, a drug that impacts a crucial protein involved in multiple PPIs could trigger wide-ranging effects that current models fail to predict.
To achieve this integration, researchers can access existing databases and tools that document PPIs. Resources like the STRING database and BioGRID offer extensive datasets on known and predicted PPIs. By merging this data with existing drug-protein interaction datasets, AI/ML models could produce more comprehensive predictions, ultimately reducing the risk of adverse drug interactions.
Developing models that effectively integrate protein-protein interactions (PPIs) into AI/ML frameworks for predicting polypharmacy side effects involves a multi-faceted approach that combines advanced computational methods with extensive biological data.
a) Data Integration and Preprocessing:
The success of these models depends on effectively merging various types of data, including drug-drug interactions (DDIs), drug-protein interactions (DPIs), and PPI networks. One of the main challenges is dealing with the diversity of data sources. While DDIs and DPIs are typically available in well-organized databases like DrugBank and PDID. PPIs are often gathered from platforms such as STRING, BioGRID, and IntAct.
Read more: https://www.pharmafocuseurope.com/information-technology/from-data-to-decision
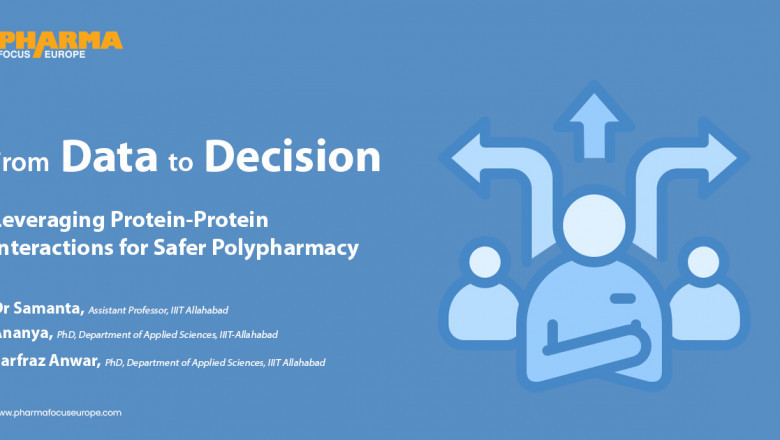

Comments
0 comment