views
A/B testing is a widely used technique in the field of data science for evaluating the effectiveness and performance of models and strategies in real-world scenarios. It provides a controlled framework for comparing two versions of a model, algorithm, or system component to determine which one performs better according to predefined metrics. A/B testing enables data scientists to make data-driven decisions and validate hypotheses with statistical rigor, ensuring that changes made to models or systems lead to measurable improvements. Its primary appeal lies in its simplicity and clarity—by splitting users or data into two groups, with each exposed to a different version of a model (A being the control and B the variant), practitioners can isolate the impact of any change and make informed choices based on observed outcomes. Data Science Interview Questions
One of the most significant benefits of A/B testing in model evaluation is the ability to understand real-world model performance. While offline metrics such as accuracy, precision, and recall provide insight into how a model behaves on historical or test data, they may not always reflect how the model will perform when deployed. A/B testing addresses this limitation by allowing models to be tested in production environments on live data, thereby capturing user behavior, feedback loops, and contextual factors that are often missing from static datasets. This real-time evaluation offers a more holistic view of model efficacy and helps identify any unanticipated effects of deploying new versions.
Another essential benefit is that A/B testing minimizes the risks associated with model updates or changes. In many business applications, even a small change in a model’s behavior can lead to significant consequences—positive or negative. By gradually rolling out a new model to a subset of users and monitoring its performance against the existing model, organizations can mitigate the risk of widespread failure. If the new model underperforms, it can be quickly rolled back without affecting the entire user base. Conversely, if it shows improvement, it can be confidently deployed to a broader audience. This controlled experimentation framework creates a safety net that promotes innovation without sacrificing stability. Data Science Career Opportunities
A/B testing also fosters a culture of continuous improvement and hypothesis testing within data science teams. It encourages data scientists to ask meaningful questions about their models and design experiments to test specific aspects, such as changes in feature engineering, tuning of hyperparameters, or incorporation of new data sources. Instead of relying on assumptions or intuition, data scientists can rely on A/B test results to guide decision-making, leading to models that are not only technically sound but also practically effective. This iterative cycle of testing, learning, and refining ensures that models evolve in alignment with business goals and user expectations.
Furthermore, A/B testing supports objective evaluation by grounding comparisons in statistically significant results. In the absence of controlled experimentation, it is often difficult to attribute improvements to specific changes, especially in dynamic environments where multiple variables influence outcomes. A/B testing isolates the variable under investigation and uses statistical methods to determine whether observed differences are likely due to the change or simply random variation. This level of rigor is critical for maintaining trust in model evaluation processes and avoiding costly missteps based on spurious findings.
In data science, A/B testing also enhances collaboration between technical and non-technical stakeholders. The results of an A/B test are typically easy to interpret and communicate, as they are framed in terms of real-world impact metrics such as conversion rate, click-through rate, revenue, or user retention. This transparency enables business leaders, product managers, and other stakeholders to understand the rationale behind model decisions and to participate meaningfully in discussions about trade-offs and priorities. By aligning model evaluation with business outcomes, A/B testing bridges the gap between data science and strategic decision-making. Data Science Course in Pune
Moreover, A/B testing enables personalization and user-centric development. By testing different models or configurations for different segments of users, organizations can learn how diverse groups respond to various approaches. This insight can inform the development of specialized models tailored to specific user needs, thereby enhancing user satisfaction and engagement. A/B testing thus becomes a powerful tool for optimizing experiences across heterogeneous user bases, ensuring that data science efforts translate into tangible value for different demographics.
The scalability of A/B testing is another noteworthy advantage. Modern data infrastructure and experimentation platforms allow for large-scale A/B tests across millions of users and numerous variations. This scalability means that data scientists can explore a broad space of model alternatives simultaneously and quickly converge on optimal solutions. Additionally, many platforms support multi-armed bandit extensions of A/B testing, where traffic is dynamically allocated to better-performing versions, further accelerating the experimentation process while maintaining statistical validity.
A/B testing also plays a pivotal role in ethical model evaluation. With growing concerns around bias, fairness, and unintended consequences in data science applications, A/B testing can be used to empirically assess how different models affect various user groups. If a new model performs better overall but disproportionately harms a particular segment, A/B testing can reveal this discrepancy before full-scale deployment. This awareness empowers organizations to design fairer, more inclusive models and to uphold ethical standards in their data science practices. Data Science Classes in Pune
Despite its many advantages, effective A/B testing requires careful planning and execution. Tests must be properly randomized, sample sizes must be sufficient to detect meaningful differences, and confounding variables must be controlled. Data scientists must also guard against issues such as peeking (prematurely ending a test based on interim results) and p-hacking (manipulating data or metrics to achieve statistically significant outcomes). These pitfalls underscore the importance of methodological rigor and domain expertise in designing and interpreting A/B tests.
In conclusion, A/B testing is an indispensable technique in data science for evaluating models in a robust, transparent, and risk-aware manner. By facilitating real-world performance validation, reducing deployment risks, encouraging empirical learning, and aligning model evaluation with business goals, it empowers data scientists to build better models and deliver greater impact. As the field of data science continues to evolve and influence every aspect of modern life, the role of A/B testing in ensuring responsible, effective, and user-centered model development will only become more critical. With its strong foundation in statistical principles and its adaptability to complex environments, A/B testing remains one of the most powerful tools for advancing the science and practice of data-driven decision-making.
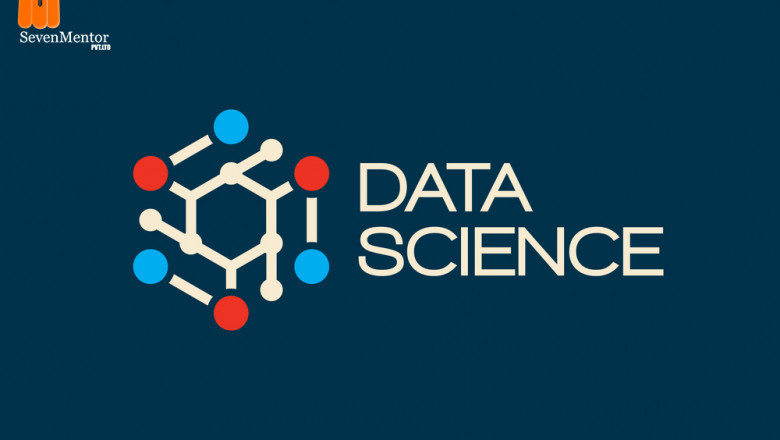

Comments
0 comment