views
Modern telecommunications rely heavily on the efficient transformation and recovery of data, a process fundamentally governed by encoding and decoding. As the industry accelerates toward 6G networks, ultra-low latency, and AI-powered infrastructures, the rise of neural and algorithmic methods is becoming increasingly pivotal. These advanced models are revolutionizing how digital signals are processed, compressed, transmitted, and interpreted across communication channels.
Introduction to Encoding and Decoding
Encoding and decoding are foundational to all forms of digital communication. Encoding converts information into a format suitable for transmission, while decoding reverses this process at the receiving end. In modern telecommunications, the demand for faster, more reliable, and more secure communication has pushed traditional models beyond their limits. Neural networks and algorithmic approaches now offer adaptive, efficient solutions that learn and evolve with data patterns.
The Evolution of Encoding and Decoding Techniques
Classical methods such as Huffman coding, LDPC (Low-Density Parity-Check), and convolutional codes once defined industry standards. However, these static models are now being augmented or replaced by intelligent systems. These newer systems are data-driven and can dynamically adjust their strategies to improve performance, especially in noisy or bandwidth-constrained environments.
Neural Network Models in Telecommunications
Neural models are transforming encoding and decoding through deep learning architectures like autoencoders, RNNs, and transformers. These systems can compress and reconstruct signals with remarkable fidelity, often surpassing traditional algorithms in efficiency. Their strength lies in learning representations directly from raw data, allowing them to adapt to changing channel conditions and interference without manual tuning.
Algorithmic Advances in Encoding and Decoding
Beyond neural models, algorithmic innovations such as turbo coding, polar codes, and iterative decoding have enhanced reliability and error correction. These models optimize latency and throughput, especially in real-time streaming and IoT applications. By using mathematical principles to maximize signal integrity, these algorithms remain essential in the layered structure of modern communication protocols.
Hybrid Approaches for Real-Time Data Optimization
A promising direction is the fusion of neural and traditional algorithmic methods. These hybrid systems leverage the interpretability of algorithms with the adaptability of neural networks. For instance, AI-assisted decoding modules can predict error patterns that traditional systems miss, resulting in seamless and faster transmission—particularly valuable in edge computing and mobile networks.
Impact on 5G and Future 6G Networks
As 5G deployment continues and 6G planning accelerates, advanced models of encoding and decoding are critical to meeting performance benchmarks. The massive device connectivity, ultra-low latency, and high data throughput requirements necessitate smarter communication protocols. Neural-algorithmic models play a central role in meeting these challenges, enabling adaptive encoding strategies and real-time decoding under unpredictable network conditions.
Challenges and Considerations for Implementation
Despite their potential, these models face implementation hurdles. Neural networks require large datasets and computational resources, making them less feasible for low-power devices. Algorithmic complexity can also increase latency if not optimized. Ensuring interoperability, minimizing power consumption, and maintaining security remain ongoing priorities for developers and researchers.
Future Prospects in Encoding-Decoding Models
The future of encoding and decoding in telecommunications lies in continued convergence. With AI at the edge, quantum communication developments, and decentralized networks, there’s a strong shift toward models that are both smart and scalable. As research deepens, models will become increasingly tailored to context—learning user patterns, network behaviors, and even adjusting in real time to environmental noise.
For more info visit https://bi-journal.com/neural-algorithmic-models-encoding-decoding-telecom-2025/
Conclusion
Neural and algorithmic models of encoding and decoding in modern telecommunications are not just theoretical innovations—they are the backbone of evolving network architectures. By combining adaptability, efficiency, and speed, they ensure communication systems are robust and future-ready. As technology advances, these models will continue to redefine how we transmit and receive data in an increasingly connected world.

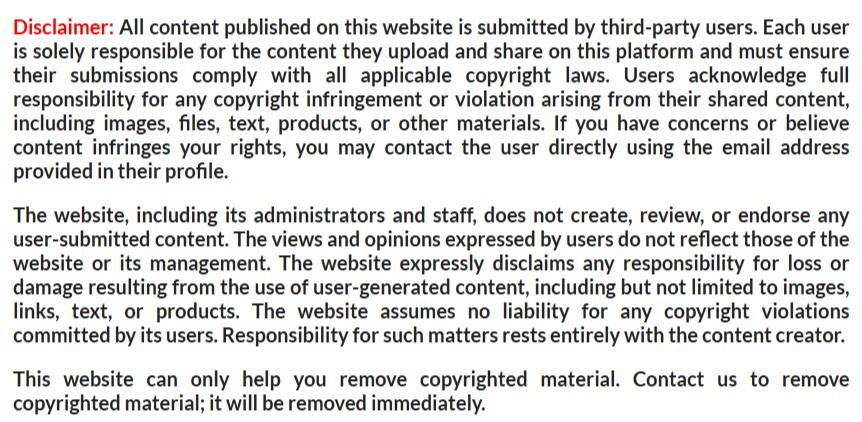
Comments
0 comment